Paper Accepted at HRI 2024: Enhancing Safety in Learning from Demonstration Algorithms via Control Barrier Function Shielding.
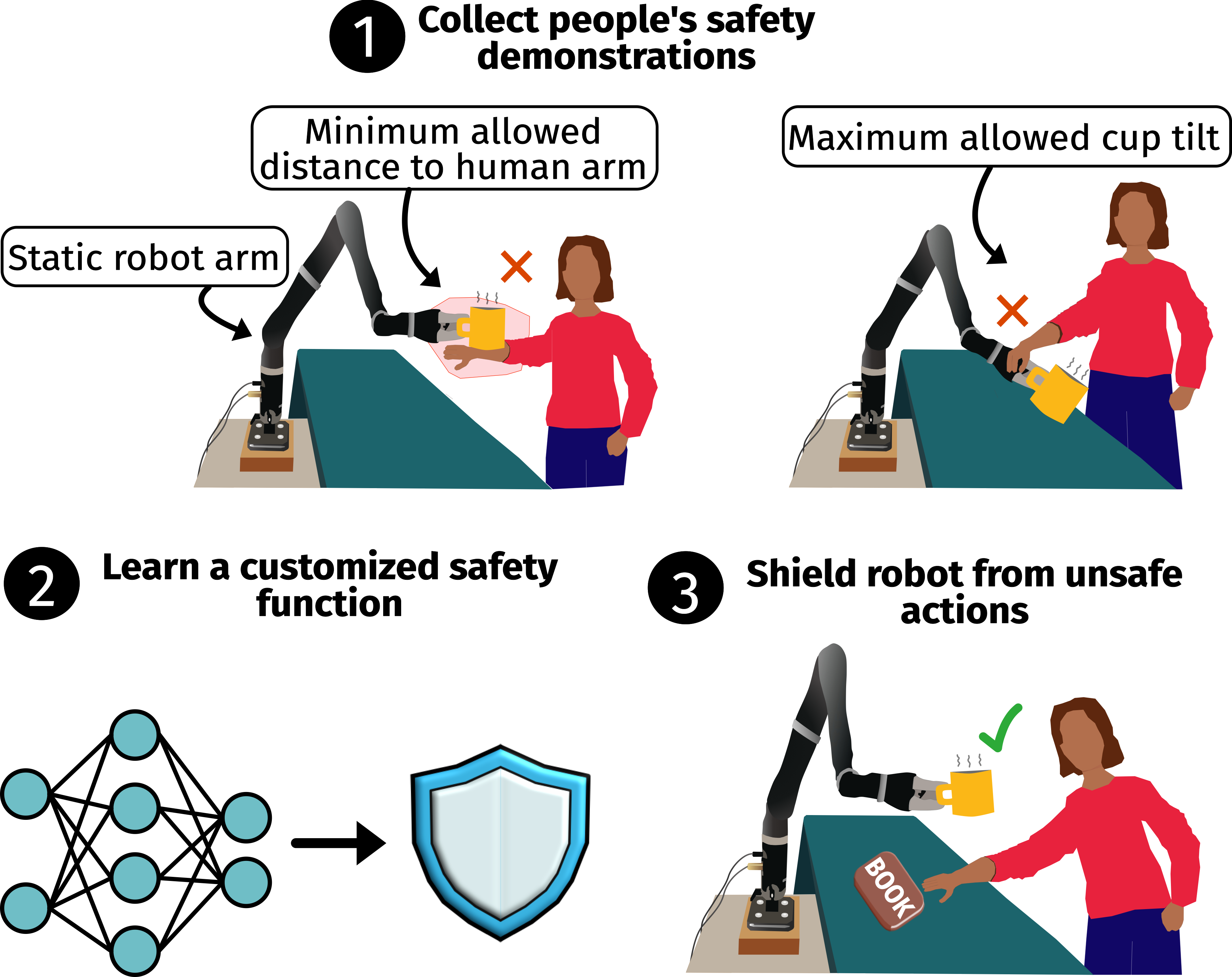
Proud of Yue Yang, Letian (Zac) Chen, Zulfiqar Zaidi, and the team for our work entitled “Enhancing Safety in Learning from Demonstration Algorithms via Control Barrier Function Shielding”. This work was presented at HRI 2024!
Full reference details: Yang, Y., Chen, L., Zaidi, Z., van Waveren, S., Krishna, A., & Gombolay, M. “Enhancing Safety in Learning from Demonstration Algorithms via Control Barrier Function Shielding,” in ACM/IEEE International Conference on Human-Robot Interaction (HRI), 2024.
Summary: We propose a new framework, ShiElding with Control barrier fUnctions in inverse REinforcement learning (SECURE), which learns a customized Control Barrier Function (CBF) from end-users that prevents robots from taking unsafe actions while imposing little interference with the task completion. We evaluate SECURE in three sets of experiments. First, we empirically validate SECURE learns a high-quality CBF from demonstrations and outperforms conventional LfD methods on simulated robotic and autonomous driving tasks with improvements on safety by up to 100%. Second, we demonstrate that roboticists can leverage SECURE to outperform conventional LfD approaches on a real-world knife-cutting, meal-preparation task by 12.5% in task completion while driving the number of safety violations to zero. Finally, we demonstrate in a user study that non-roboticists can use SECURE to effectively teach the robot safe policies that avoid collisions with the person and prevent coffee from spilling.